Looking for something?
Could Artificial Intelligence Provide the Key to Life Saving Medicines?
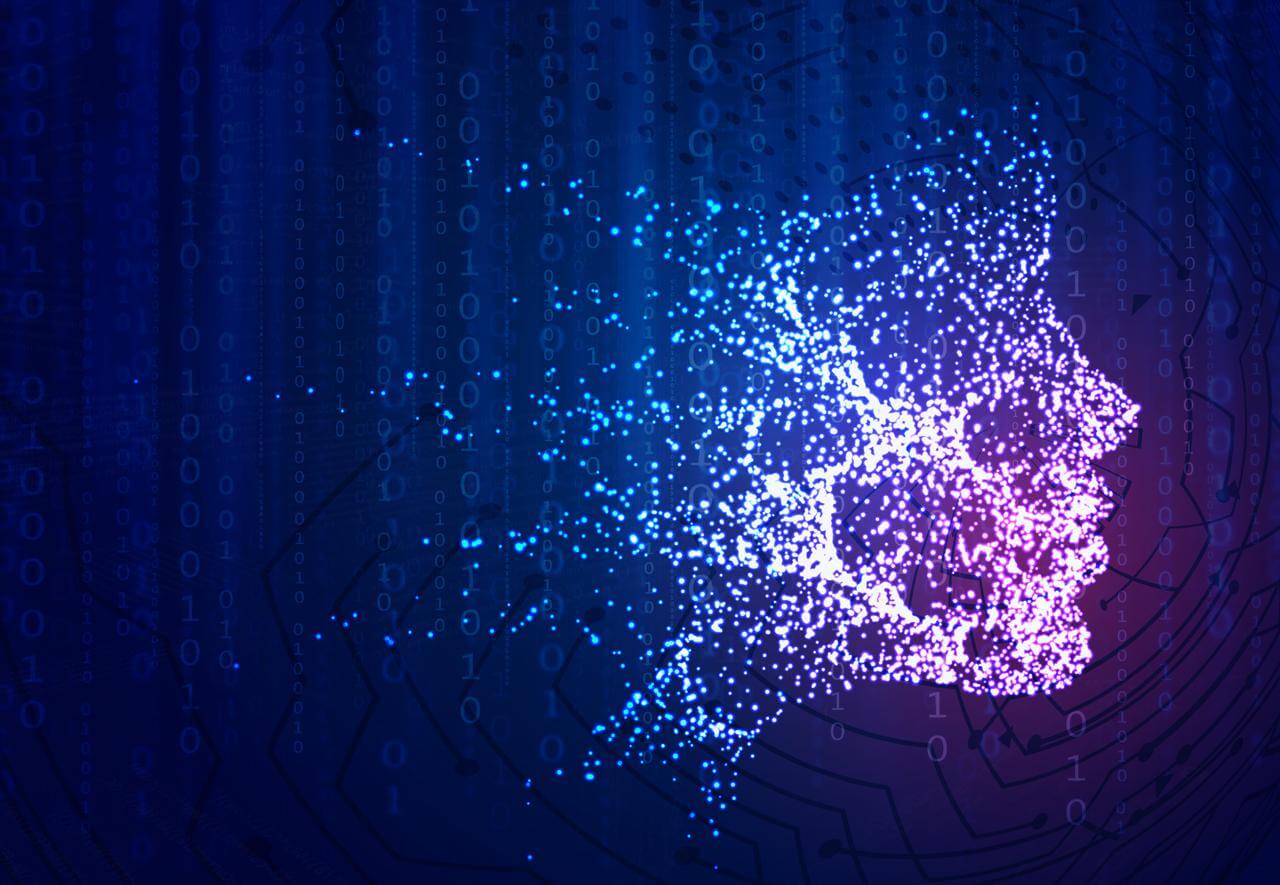
Drug discovery is time consuming and expensive, requiring trial and error screening. This means that many people have to wait decades, or a lifetime, to find a treatment for their disease. Others, especially those with rare diseases, may never have hope of a treatment. However, AI and deep learning have been used increasingly in biological research and biomedical applications and there is a growing emergence of companies and academic labs trying to harness AI to predict oligonucleotides and other peptide-based molecules to improve on traditional trial-and-error screening methods.
AI is likely to be a vital part of drug development in the future for many reasons. For example, the earlier a genetic disease is discovered and treated, the lower impact it potentially has on a person’s health, wellbeing, and life expectancy. If a genetic disease could be discovered and medicine created to provide treatment long before enough damage is done to cause symptoms, it is theoretically possible that the person will never actually develop symptoms and live a disease free life.
However, the sheer amount of data that must be compiled and interpreted makes these tasks very difficult, if not impossible, for the unaided human mind.
AI and computational modeling can analyze enormous quantities of data, make inferences, and deliver insights that humans are unable to (1). This could allow AI to deliver results that include discovering underlying genetic causes of disease through widespread screening and predicting the sequence most likely to provide therapeutic effect, as well as designing the safest, most effective therapeutic that can be delivered to the affected cells or tissue.
Michael Wainberg et al. published a Perspective titled “Deep learning in biomedicine” in which they provide information about machine learning and deep learning applications to biology and medicine. They explain that deep learning can be performed with a deep neural network (DNN), which is composed of layers and the outputs of one layer feed into the inputs of the next, allowing complex input-output relationships. The stacks of transformations are extremely powerful, flexible, and trainable.
Wainberg provides an interesting example to illustrate an important strength of deep learning: its ability to use intermediate variables for different but related tasks. For example, a hypothetical intermediate variable that detects the presence of an RNA secondary structure could be used in subsequent layers to detect a protein–RNA interaction, a microRNA target or the formation of a splicing lariat (1).
A few notable applications of deep learning are particularly relevant to our field (1):
- Deep learning is well suited to modeling the molecular phenotypes of genetic variants impacting transcription, splicing, transcript stability and translation regulation.
- Deep learning is critical in constructing quantitative models of molecular phenotypes, interfacing DNA sequence to the growing body of molecular activity data produced using next generation sequencing and other technologies.
- Deep Neural Networks (DNNs) can be trained using the reference genome sequence and annotations (for example, known splice sites) or molecular profiles (for example, exon inclusion in cell types of interest). Using a technique known as in silico mutagenesis, these reference data models can then be used to predict the effect of genetic variants.
Deep models can go wrong in many ways, and certain challenges will need to be addressed such as data mismatch, selection bias, and target mismatch, to name just a few (1). In order for human experts to rely on AI and machine learning, we must first create AI that produces nearly error-free results.
Currently, AI is not 100% predictive and is only as good as the data mined. There are an astonishing number of factors and properties of a molecule that go into selecting it and moving it forward into the clinic, and AI needs to be created that takes all these into account.
An additional challenge lies in the fact that larger amounts of data are generally required to accurately train a DNN. However, the production of large-scale biomedical databases is rapidly increasing. Additionally, “in data-limited situations, deep learning is well suited to leverage large datasets on related problems to improve performance, in an approach called transfer learning, and with large enough datasets the performance of deep learning is unparalleled” (1). Deep learning can also naturally integrate input data from multiple modalities and targets from multiple tasks (1).
While these systems do still require some level of coding by scientists, it is likely that in the near future, AI will propose the models and then systematically evaluate them, all with cloud computing (1).
Many scientific teams are developing and using AI and machine learning to improve human health.
Deep Genomics uses their AI workbench to analyze enormous amounts of in vitro and in vivo data to find quicker paths to solutions. It begins with target discovery, which includes identifying disease-causing mutations and ways of fixing the problem that results from the mutation. Then, their AI can swiftly assess millions of different potential targeted therapies that are likely to produce the best results. The platform can “successfully predict alterations in molecular phenotypes, such as transcription, splicing, translation and protein binding.”
Of course, this requires an extensive library of data. GenomeKit is a proprietary library that Deep Genomics created to provide fast and easy access to genomic resources including sequence, data tracks, and annotations and it also works with genome variants.
Data on every compound identified using the platform is collected, from therapeutic candidates to novel exploratory compounds. Their platform also uses public datasets and recently published discoveries are evaluated and rapidly incorporated. Currently, their focus is on developing steric blocking oligonucleotides.
TargetRanch is a software system developed by Deep Genomics that uses artificial intelligence (AI) predictors trained on large-scale genomics datasets to identify disease causing mutations – and oligonucleotide therapeutics that could treat the resulting problem. The potential was realized when a variant in the ATP7B gene that causes Wilson disease was identified, along with the idea that the variant may be pathogenic due to an RNA splicing alteration (2). Follow-up experiments validated the prediction and, using the same AI predictive models, they designed an oligonucleotide that corrects the splicing and brings ATP7B function levels back to normal.
Founder and CEO, Brendan Frey, stated that “For novel target mechanisms identified by our AI Workbench, 50% of them result in lead drug candidates, and we can achieve that within 12 months.”
Other current disease targets include more effectively suppressing urate synthesis to treat Gout, restoring NPC1 protein levels and function to treat Niemann-Pick disease type C, and restoring Granulin Precursor (GRN) protein levels and function to treat Frontotemporal Dementia caused by partial loss of GRN function due to dominant pathogenic variants in the gene Granulin Precursor.
Deep Genomics is also collaborating with BioMarin to discover and develop oligonucleotide medicines to treat rare diseases. Deep Genomics is using their platform to identify and validate target mechanisms and lead candidates, then BioMarin will advance these compounds into preclinical and clinical development.
Creyon Bio is using a machine learning and AI-backed platform to engineer safe, on-demand oligonucleotide medicines. This approach should provide predictable safety and performance before ever being tested for “modalities ranging from single-stranded antisense oligonucleotides (ASOs) that reduce gene expression levels or change splicing events to small interfering RNA (siRNA), to DNA and RNA editing systems, to even targeting aptamers.”
From the sequence of the oligonucleotide to the sugar, nucleobase, and backbone chemistries, the platform has the potential to calculate the billions of possible designs to efficiently develop predictive models to produce safe and effective medicines. As if that weren’t ambitious enough, they are also engineering delivery systems that target specific cell types and tissues.
Creyon is currently involved in preclinical research and collecting extensive data from in vivo, in vitro, and ex vivo experiments, drawing from data that have proven successful in prior research.
They are not focused on a single drug, but rather on “uncovering the design rules and engineering principles of oligonucleotide-based medicines. However, with their deliberate approach, Creyon intends to produce accurate predictions that allow for rapid, cost-effective development of treatments, whether it be an N-of-1 medicine or a treatment for millions.
PFred (PFizer RNAi Enumeration and Design tool) is a user friendly, open source software system that utilizes algorithms to assist with the entire design process of a library of siRNAs or RNase H-dependent antisense oligonucleotides for a specific gene target. Sequences are chosen by using algorithms built from sequence-activity relationships found in public datasets and internal collections. It can be customized by experienced developers and allows for the rational design of oligonucleotides that incorporate design criteria that are important for stability and potency (3).
Not only are deep learning systems being used to identify targets and drug candidates, but also to make the medicines safer and more effective.
The Pentelute Team and Gómez-Bombarelli Team at the Massachusetts Institute of Technology worked with Sarepta Therapeutics to create Peptimizer, a neural network that uses machine learning algorithms to optimize cell penetrating peptides (CPPs). The teams used this model to predict nontoxic miniproteins that efficiently deliver antisense cargo in mice. The CPPs improve the delivery of oligonucleotides into cells 20 to 50-fold in cell assays. Not only were the CPPs effective in mice, but they also helped the drugs reach the heart (4).
Peptimizer can be used to design different molecule shapes in addition to simple straight CPPs, is applicable to any biological polymer, and is able to predict activities beyond the training dataset (4).
Delivery of oligonucleotides to various tissues has been one of the major challenges in advancing oligonucleotide drugs. If artificial intelligence could not only discover solutions but also rapidly find the most efficient and least toxic method of delivery, it would exponentially increase the potential of developing new drugs to treat disease.
QLattice is a machine learning model that “searches among thousands of potential models for the one graph with the right set of features and interaction combinations that, in conjunction, unfolds the perfectly tweaked model to your problem.” In addition to a highly accurate model, you also have a simple visual depiction to see how data is manipulated to deliver the predictions.
QLattice was used to find a mathematical expression to serve as a hypothesis to determine “What features of an ASO contribute to toxicity?” It was determined that LNA load in the ASO contributed to toxicity. Additionally, the amount of LNA modifications in the 5′ flank had a stronger effect on its toxicity than the number of modifications in the 3′ flank. With the ability to create a specific hypothesis based on the data provided, QLattice could easily be used to develop oligonucleotides that are less toxic.
Since a key challenge in designing oligonucleotides is the potential for toxicity, especially at high doses, using machine learning to mitigate this challenge would be an enormous benefit to oligonucleotide medicines.
Vesalius Therapeutics was recently launched with the goal of determining underlying causes of illnesses, such as type II diabetes, heart disease, and Alzheimer’s Disease, in which a broad range of patients are given a single diagnosis but actually are due to many different biological and/or genetic causes. Vesalius’ Diamond technology platform combines data from large clinical databases, genetics and genomics information, artificial intelligence and machine learning, and proprietary experimental models which will be used to find defined subpopulations of patients and create an effective treatment.
Other companies that we have mentioned in previous posts are incorporating machine learning into their platforms with great success.
Alltrna is developing a platform utilizing machine learning to explore tRNA biology to eventually use tRNA as a programmable medicine.
Envisagenics uses artificial intelligence, RNA sequencing data, and high performance computing in their SpliceCore platform to predict and discover new splicing errors and swiftly design therapeutic compounds to correct them.
Gritstone Bio uses Gritstone Edge, their proprietary AI-based platform to understand which antigens and neoantigens will be transcribed, translated, processed, and presented on a cell surface by human leukocyte antigen (HLA) molecules, making them visible to T-cells. This is done to find targets for immunization to develop cancer immunotherapies.
Compared to the science fiction vision of an AI that can nearly relate as a human, with god-like intelligence, our current Artificial Intelligences are barely in their infancy. Even a more feasible AI that can problem solve with the insight, efficiency, and accuracy of a human is likely to still be years away. While we are far from creating AI that can truly discover underlying causes of disease and design an oligonucleotide therapeutic with minimal human effort, it is exciting that companies and investors are starting to mine all of the existing knowledge that oligonucleotide companies like Ionis and Alnylam have laid the foundation with, to improve on practices in drug design and discovery. It is fascinating to watch as drug discovery of the future emerges.
References:
- Wainberg, M., Merico, D., Delong, A. et al. Deep learning in biomedicine. Nat Biotechnol 36, 829–838 (2018). https://doi.org/10.1038/nbt.4233
- Merico D, Spickett C, O’Hara M, Kakaradov B, Deshwar AG, Fradkin P, Gandhi S, Gao J, Grant S, Kron K, Schmitges FW, Shalev Z, Sun M, Verby M, Cahill M, Dowling JJ, Fransson J, Wienholds E, Frey BJ. ATP7B variant c.1934T > G p.Met645Arg causes Wilson disease by promoting exon 6 skipping. NPJ Genom Med. 2020 Apr 8;5:16. doi: 10.1038/s41525-020-0123-6. PMID: 32284880; PMCID: PMC7142117.
- Sciabola S, Xi H, Cruz D, Cao Q, Lawrence C, Zhang T, Rotstein S, Hughes JD, Caffrey DR, Stanton RV. PFRED: A computational platform for siRNA and antisense oligonucleotides design. PLoS One. 2021 Jan 22;16(1):e0238753. doi: 10.1371/journal.pone.0238753. PMID: 33481821; PMCID: PMC7822268.
- Schissel CK, Mohapatra S, Wolfe JM, Fadzen CM, Bellovoda K, Wu CL, Wood JA, Malmberg AB, Loas A, Gómez-Bombarelli R, Pentelute BL. Deep learning to design nuclear-targeting abiotic miniproteins. Nat Chem. 2021 Oct;13(10):992-1000. doi: 10.1038/s41557-021-00766-3. Epub 2021 Aug 9. PMID: 34373596; PMCID: PMC8819921.
Disclaimer:
“The views, opinions, findings, and conclusions or recommendations expressed in these articles and highlights are strictly those of the author(s) and do not necessarily reflect the views of the Oligonucleotide Therapeutics Society (OTS). OTS takes no responsibility for any errors or omissions in, or for the correctness of, the information contained in these articles. The content of these articles is for the sole purpose of being informative. The content is not and should not be used or relied upon as medical, legal, financial, or other advice. Nothing contained on OTS websites or published articles/highlights is intended by OTS or its employees, affiliates, or information providers to be instructional for medical diagnosis or treatment. It should not be used in place of a visit, call, consultation, or the advice of your physician or other qualified health care provider. Always seek the advice of your physician or qualified health care provider promptly if you have any healthcare-related questions. You should never disregard medical advice or delay in seeking it because of something you have read on OTS or an affiliated site.”